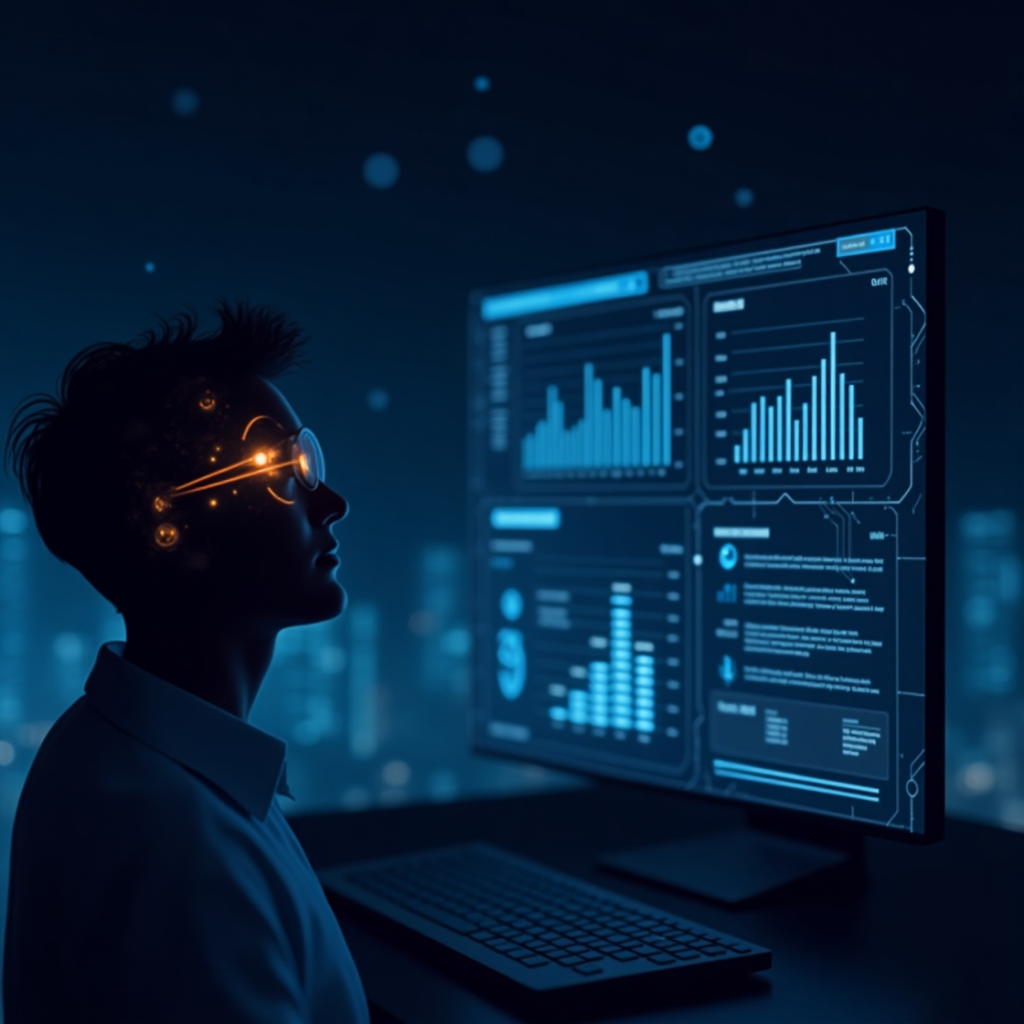
A Report Focused on Data Collection and Analysis in 2025.
This report examines the current state of AI’s integration within market research, focusing on its impact on data collection and analysis.
I. Introduction:
Market research, traditionally reliant on surveys, focus groups, and manual analysis, is evolving to embrace the power of AI. AI offers the potential to automate processes, derive deeper insights from complex datasets, and ultimately, provide businesses with more accurate and actionable market intelligence. This report explores the current key applications of AI in data collection and analysis, along with the challenges and opportunities that lie ahead.
We estimate 40-50% of market research firms are currently using AI in some capacity for data collection and analysis.
Supporting Evidence:
- Qualtrics 2025 Market Research Trends Report (https://www.qualtrics.com/ebooks-guides/market-research-trends/):
- SurveyMonkey AI in Marketing Statistics
- Backlinko Market Research Statistics
- TT Consultants 2024 Trends Report
Factors Influencing Adoption:
- Increased data volume and complexity: AI is becoming essential to handle the growing amount of data.
- Demand for faster insights: AI enables quicker analysis and speeds up decision-making.
- Improved accuracy and efficiency: AI can reduce errors and improve the quality of insights.
- Growing availability of AI tools and services: More user-friendly and accessible AI tools are emerging.
- Increased awareness and understanding of AI: More researchers are recognizing the potential of AI.
Caveats:
- Varying definitions of AI: The definition of “AI” can be broad, so reported usage might include simple automation as well as more advanced techniques.
- Differences across firm sizes and industries: Larger firms and those in data-heavy industries may have higher adoption rates.
- Ongoing evolution: AI adoption is rapidly increasing, so these estimates will likely change in the near future.
Overall, it’s safe to say that a significant portion of market research firms are already using AI, and this trend is only going to accelerate.
II. AI-Powered Data Collection:
AI is revolutionizing how market research data is gathered, moving beyond traditional methods to capture richer, more nuanced information.
A. Automated Surveys: AI-powered chatbots can conduct interactive surveys, adapting questions based on respondent answers and providing a more engaging experience. This leads to higher completion rates and more accurate data. Furthermore, natural language processing (NLP) enables chatbots to understand and respond to open-ended questions, gathering qualitative data at scale.
- https://landing.outset.ai/ An example of how AI is changing interaction with survey respondents and data collection.
B. Social Media Listening: AI algorithms can monitor social media platforms, forums, and review sites to understand consumer sentiment, identify emerging trends, and track brand perception. NLP and machine learning are used to analyze text and identify emotions, providing valuable insights into consumer opinions and preferences.
- Brandwatch: (brandwatch.com) A comprehensive platform offering deep listening, powerful analytics, and AI-powered insights. Great for large brands and agencies needing extensive data.
- Talkwalker: (talkwalker.com) Another strong contender with extensive coverage, AI-powered analytics, and features for crisis management and trend identification.
- Mention: (www.mention.com) Focuses on real-time monitoring and alerts, with an intuitive dashboard and influence scoring to identify key individuals.
C. Passive Data Collection and Behavioral Tracking: AI enables the collection of passive data through various sources, such as website analytics, mobile apps, and IoT devices. This data provides insights into actual consumer behavior, rather than relying solely on self-reported information. AI algorithms can analyze these vast datasets to identify patterns and predict future behavior.
- Convizit: (https://convizit.com/) This AI-driven platform automatically captures user events on websites and web applications, requiring no manual tagging or coding. It provides rich behavioral data streams to analytics and marketing platforms.
- Heap: (https://heap.io/) Heap automatically captures user interactions (clicks, swipes, form submissions, etc.) on websites and mobile apps. It uses AI to analyze this data and provide insights into user behavior.
- FullStory: (https://www.fullstory.com/) FullStory offers session replay and AI-powered analytics to understand user journeys and identify friction points in digital experiences.
D. Image and Video Analysis: AI-powered image and video recognition can analyze visual content to understand consumer preferences, identify product usage patterns, and track brand visibility. This is particularly useful in areas like retail research, where AI can analyze shelf layouts and customer interactions.
Cloud-Based APIs:
- Google Cloud Vision API: (https://cloud.google.com/vision) A versatile API offering image labeling, face and landmark detection, OCR, explicit content detection, and more. Great for general-purpose image analysis.
- Microsoft Azure AI Vision: (https://azure.microsoft.com/en-us/products/ai-services/ai-vision/) Similar to Google’s offering, Azure’s API provides object detection, image tagging, OCR, and even spatial analysis.
- Amazon Rekognition: (https://aws.amazon.com/rekognition/) Amazon’s image and video analysis service with features like object and scene detection, facial analysis, celebrity recognition, and content moderation.
Specialized Platforms:
- Clarifai: (www.clarifai.com) A platform for building AI-powered visual recognition models. Offers pre-trained models and tools for training custom models.
- Imagga: (www.imagga.com) Focuses on automated image tagging and categorization, making it easy to organize and search large image collections.
- CloudSight: (www.cloudsight.ai) Provides image analysis with a focus on understanding the context and meaning of images, going beyond basic object recognition.
- Sightengine: (www.sightengine.com) Specializes in image and video moderation, using AI to detect inappropriate content, nudity, and other sensitive material.
Video Analysis:
- Google Cloud Video Intelligence API: (https://cloud.google.com/video-intelligence) Analyzes video content to identify objects, scenes, and activities, making it useful for video indexing and search.
- Amazon Rekognition Video: (https://aws.amazon.com/rekognition/video/) Provides real-time analysis of video streams for tasks like object tracking, person identification, and activity recognition.
- Valossa: (www.valossa.com) Offers video analysis with a focus on understanding the emotional and narrative aspects of video content.
E. Voice of Customer (VOC) Analysis: AI can analyze voice recordings from customer service interactions, reviews, and feedback forms to understand customer needs, identify pain points, and track customer satisfaction. NLP is used to transcribe and analyze spoken language, extracting valuable insights from unstructured data.
III. AI-Powered Data Analysis:
The real power of AI in market research lies in its ability to analyze vast amounts of data and extract meaningful insights.
- A. Predictive Analytics: AI algorithms can build predictive models to forecast future market trends, consumer behavior, and product demand. This allows businesses to make proactive decisions and optimize their strategies.
- B. Customer Segmentation: AI can segment customers based on various factors, such as demographics, behavior, and preferences. This allows businesses to personalize their marketing efforts and target specific customer groups with tailored messages.
- C. Text and Sentiment Analysis: NLP techniques can analyze text data from various sources, such as surveys, social media, and customer reviews, to understand consumer sentiment, identify key themes, and extract valuable insights.
- D. Data Visualization and Reporting: AI can automate the creation of data visualizations and reports, making it easier for researchers to understand and communicate their findings. AI-powered dashboards can provide real-time insights into market trends and customer behavior.
- E. Pattern Recognition: AI algorithms can identify complex patterns in data that might be missed by human analysts. This can help businesses identify emerging trends, detect potential risks, and understand the drivers of customer behavior.
IV. Challenges and Opportunities:
While AI offers significant benefits to market research, several challenges need to be addressed:
- Data Privacy and Security: Collecting and analyzing large amounts of personal data raises concerns about privacy and security. Market research companies must ensure compliance with data protection regulations and implement robust security measures.
- Bias in AI Algorithms: AI algorithms can be biased if trained on biased data. It is crucial to ensure that data used to train AI models is representative of the target population to avoid skewed results.
- Ethical Considerations: The use of AI in market research raises ethical questions about transparency, informed consent, and the potential for manipulation. Market research companies must adhere to ethical guidelines and ensure that AI is used responsibly.
- Skills Gap: The adoption of AI in market research requires a skilled workforce that can develop, implement, and interpret AI-powered solutions. There is a need for training and education to bridge the skills gap.
Despite these challenges, the opportunities for AI in market research are immense:
Deeper Insights: AI can uncover hidden patterns and insights in data that would be difficult or impossible to detect with traditional methods.
Faster Analysis: AI can automate data analysis processes, significantly reducing the time required to generate insights.
Improved Accuracy: AI-powered analysis can be more accurate and less prone to human error than traditional methods.
Enhanced Customer Experience: AI can personalize the research experience for respondents, leading to higher engagement and more accurate data.
V. Conclusion:
AI is transforming the market research industry, empowering businesses with more accurate, timely, and actionable insights. While challenges related to data privacy, bias, and ethics need to be addressed, the potential benefits of AI are undeniable. As AI technology continues to evolve, we can expect to see even greater innovation in data collection and analysis, leading to a more data-driven and customer-centric approach to market research. Market research companies that embrace AI will be better positioned to understand consumer behavior, anticipate market trends, and drive business growth in the years to come.